From Code to Cure:
How AI is reshaping drug discovery, the UK's standing and the role of real estate.
11 minutes to read
Artificial intelligence (AI) is reshaping industries and processes, but few areas show more promise than drug discovery. With spiralling costs and lengthy timelines stalling pharmaceutical innovation, AI offers a lifeline: faster processes, lower costs, and more effective medicines.
The traditional model of drug development is anything but efficient. On average, developing a single drug costs over $2 billion[1], with a staggering 90% of candidates failing clinical trials[2]. Even when they proceed, bringing a drug to market typically takes a decade or longer.
Against this backdrop, AI is being heralded as a transformative force. Potentially reducing discovery timelines to just two years and slashing costs by up to 70%. Over the next decade, AI could contribute to discovering 50 new therapies, unlocking over $50 billion in value[3]. This is particularly significant for tackling diseases that currently lack effective treatments— only 5% of known rare diseases have a treatment[4].
A new toolkit for discovery
At its core, AI in drug discovery leverages vast datasets— including genomic information, molecular structures, and clinical trial outcomes—to identify potential drug candidates, predict their efficacy, optimise their design, and streamline the approval process.
While computational methods in drug discovery have become widely adopted, the use of AI, particularly generative AI, is a more recent development. Unlike traditional AI models that classify or predict, generative AI models produce new outputs.
AI-powered drug discovery is a subset of TechBio. TechBio refers to any company or research initiative that uses cutting-edge technology to advance biological sciences. At the same time, AI-powered drug discovery focuses on AI applications within the drug development process.
The potential applications are wide-ranging. AI accelerates areas that include target identification, sifting through large complex biological data to pinpoint disease-associated molecules. In lead optimisation, AI techniques are employed to screen vast compound libraries and optimise lead compounds, streamlining the drug discovery process. AI also forecasts drug properties, streamlining formulation. Beyond this, it offers novel insights into drug repurposing, uncovering new uses for existing medicines, and simplifying the clinical trial and regulatory approval process.
Example use cases:
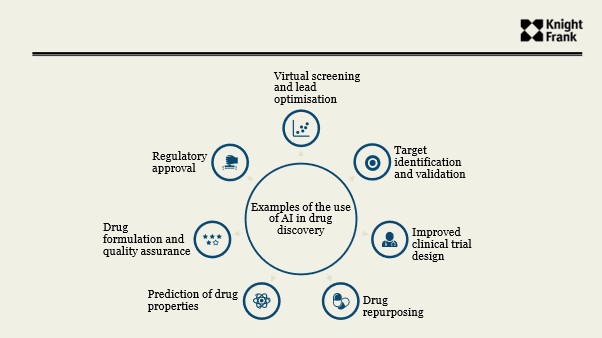
AI capabilities are advancing rapidly, with the next significant phase expected to be general or strong AI. These systems will demonstrate greater autonomy, adapt to various contexts, and not be confined to specific tasks. Looking further ahead, the development of AI systems surpassing human intelligence or even the collective intelligence of humanity may become a possibility in the distant future.
The complex web of companies
The field of AI-powered drug discovery is diverse. Within this burgeoning field, firms occupy niches across a broad spectrum from comprehensive, end-to-end solutions providers to specialised players focused on specific parts of the value chain. The size and maturity of these firms also vary, from agile startups to established pharmaceutical and technology behemoths. Many new startups are charting their own paths, developing proprietary drug pipelines, or out licencing to pharma companies. Meanwhile, collaborations between tech companies, startups, and pharma giants abound.
A thriving ecosystem supports growth, drawing from the combined efforts of contract research organisations, academic institutions, research bodies, venture capitalists, and regulatory agencies, amongst other stakeholders.
Moreover, the sector's influence extends beyond its core focus. It converges with adjacent fields such as diagnostics, wearables, and quantum computing. It helps drive the growth of infrastructure providers such as data centres.
Example companies:
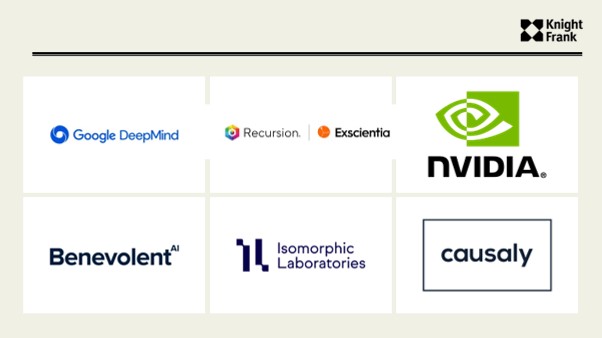
Signs of progress
Recent examples highlight AI's growing influence. In 2023, Insilico Medicine used its generative AI platform to design a drug targeting idiopathic pulmonary fibrosis, advancing it from hypothesis to preclinical validation in under 18 months. In May 2024, Google DeepMind launched AlphaFold 3, the latest version of its groundbreaking model. While earlier versions focused on predicting a protein's 3D structure from its amino acid sequence, AlphaFold 3 goes further, predicting the structures of nearly all biological molecules and modelling interactions between them. This is the first system capable of accurately predicting interactions between such a wide range of molecules.
Recent statistics highlight the rapid growth and increasing momentum of AI-powered drug discovery. Over the past five years, publications and patents in this field have surged by 34% and 17% annually, reflecting a sharp uptick in innovation and research activity. Corporate interest has also followed suit. Mentions of AI in pharmaceutical financial statements skyrocketed from a modest 55 in 2016 to an impressive 751 in 2024, while the number of news articles mentioning pharma companies and AI reached a record 1,646 in 2024 compared to 1,305 in 2023[5]. GlobalData's drug database reports that over 3,000 drugs are being developed or repurposed using AI. Oncology is the leading therapy area for drugs under development, followed by central nervous system and immunology.
Growth is being turbocharged by funding, technological advancements, increased availability of large biomedical datasets, strategic partnerships and regulatory and government support in specific locations.
However, the road ahead will not be without setbacks. Trust in the technology remains fragile, compounded by regulatory hurdles, data privacy concerns, and a shortage of high-quality datasets despite improvements. The interdisciplinary expertise required to bridge biology and computational science is in short supply, leaving critical gaps in the workforce. Meanwhile, high implementation costs and outdated legacy systems further delay adoption. For large companies' organisational inefficiencies and cultural resistance add another layer of complexity.
As companies and other key stakeholders grapple with challenges, the field will likely experience growing pains before reaching its full potential. In our view, big pharma will gain the most from AI in the near term, particularly in areas such as drug repurposing.
Britain's edge
The UK is carving out a leading role in AI-powered drug discovery, leveraging its renowned research institutions, leading global data sets, and vibrant life sciences and AI clusters. It is home to 79 companies at the forefront of AI adoption in the pharmaceutical sector, placing it fourth globally and first in Europe, trailing only the United States, China, and South Korea. According to Nature.com, the UK is third behind the US and China in the number of citations in computational biology and bioinformatics.
This intellectual heft is matched by financial clout. Britain ranked second to the US in capital invested for AI-driven drug discovery firms over the past five years, underscoring its appeal to investors. London, the epicentre of this activity, has emerged as a global hub. Over the same five-year period, it has been the leading city globally regarding the number of deals. Nearly half of the UK's AI-focused pharma companies are based in the capital[6]. Several AI hubs have been launched in London. GSK and Novo Nordisk have set up shop in the city's Knowledge Quarter, with GSK investing £10 million in its AI-focused research centre. Recursion, a US-based biotech company, recently acquired office space there. Recursion's CEO, Chris Gibson, praised London's unique strengths: "The UK seems to have done the best job in the world of educating people at the intersection of technology and biosciences."
London's academic institutions are also stepping up. University College London (UCL) has launched a new AI hub, while Imperial College and the University of Liverpool are spearheading efforts to develop AI tools for chemistry. Queen Mary University of London, for its part, is partnering with Exscientia and others to train a new generation of AI-savvy researchers alongside other initiatives.
Top ten cities by HQ location of AI in Pharma leaders
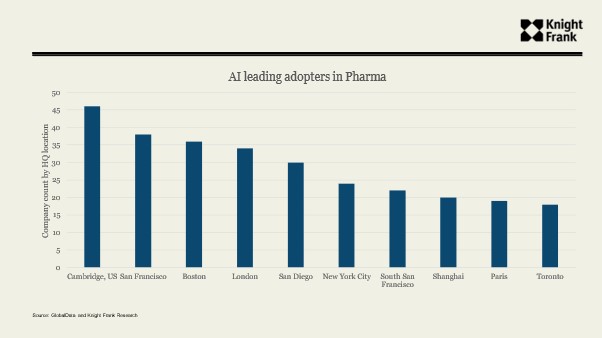
AI's operational and real estate impact
The adoption of AI in drug discovery is not just reshaping science but also forcing fundamental changes across operations, talent strategies, and real estate decisions. As the technology matures, it drives process reengineering, corporate restructuring, and new skills requirements, fosters mergers and acquisitions, spurs strategic partnerships and boosts outsourcing. At the same time, a wave of new entrants—ranging from nimble startups to tech giants —is reshaping the competitive landscape.
Reimagining talent strategies
Talent is becoming a decisive factor for companies navigating the convergence of AI and life sciences. While traditional expertise in the "Golden Triangle" of London, Oxford, and Cambridge remains critical, computational talent is in high demand. Analysing the top skills among professionals in the leading AI-powered drug discovery companies reveals that London is once again in pole position, home to nearly a third of the nationwide talent pool. The other talent hotspots are Cambridge, Manchester, and Oxford. Scotland also deserves a mention, with 17,700 professionals based across Edinburgh and Glasgow who have these skills.[7]
Companies are addressing gaps by hiring and upskilling existing employees to bridge science and AI capabilities. AstraZeneca, for example, has launched an AI accreditation programme for its staff. Learning experiences range from online learning to keynote seminars, lab sessions, reflection activities, and strategic thinking workshops.
Real estate decisions for those embedded in this sector will be driven by the need to be near hubs of computational expertise, ensuring access to top talent. At the same time, facilities must be designed to attract and retain highly sought-after professionals, blending functionality with the amenities and workspaces expected in a competitive talent market. There may also be a heightened requirement for spaces for learning.
While it is too soon to say with certainty what impact AI will have on headcount, available forecasts expect AI to automate up to 25% of drug discovery roles and 21% of clinical research jobs. However, the technology will also reshape existing roles and create new ones. Real estate decision-makers must work with other departments, such as HR, to map workforce tasks, identify those most at risk of automation, and plan for future workforce requirements. Adaptable real estate is critical.
Partnerships and Collaboration
Strategic partnerships are pivotal in accelerating AI adoption within drug discovery. Leading pharmaceutical companies, tech firms, and specialised organisations are joining forces to leverage their respective strengths, with collaboration becoming the hallmark of this nascent field.
OpenAI, for example, has partnered with Sanofi to create custom AI tools to streamline drug development processes. AstraZeneca, meanwhile, has teamed up with BenevolentAI and Illumina to apply AI to genomic analysis and patient stratification, enhancing clinical trial precision. Novartis has forged alliances with Microsoft, Anumana, and Deciphex to advance AI's role in detecting cardiovascular diseases, designing clinical trials, and improving preclinical evaluations. Isomorphic Labs, a sister company of DeepMind, has struck deals with Eli Lilly and Novartis worth up to $3 billion.
Stakeholders are also joining forces to develop data platforms. Open Targets, for example, is a platform founded in 2014 as a public-private partnership. It uses human genetics and genomics data for drug target identification and prioritisation. Partner institutions include The European Bioinformatics Institute, Genentech, GSK and The Wellcome Sanger Institute.
Real estate must evolve to meet the demands of AI-driven drug discovery partnerships. Location decisions will increasingly prioritise areas offering a robust collaborative partner ecosystem.
A new wave of entrants
New players are transforming the sector. Exscientia, once a 75-employee startup, has grown into a 500-strong global leader, leveraging AI for precision drug discovery. Tech companies like Nvidia are also making significant inroads, developing AI models like BioNeMo and investing heavily in startups like Genesis Therapeutics and Generate Biosciences.
These firms require advanced labs for both wet and dry work and high-performance computing facilities for secure data processing. Modular lab designs and adaptable office spaces are becoming essential to accommodate growth and evolving technological needs.
Needs will also vary depending on the specific niche. For instance, those involved in clinical trials will likely transition from large, centralised facilities to distributed networks as trials become increasingly decentralised via technology and data. Furthermore, AI can be crucial in analysing extensive datasets to determine ideal trial locations based on patient demographics, disease prevalence, and healthcare access.
Mergers and acquisitions on the horizon
Mergers and acquisitions in the nascent field of AI-driven drug discovery could grow as firms seek to achieve scale in an increasingly competitive landscape. The recent merger of Recursion Pharma with Exscientia highlights this trend. At the same time, BenevolentAI's combination with Odyssey—a deal that stands as Europe's largest SPAC transaction—demonstrates how AI-focused firms leverage financial markets to accelerate growth. Large pharmaceutical companies eager to secure rights to innovative drugs or cutting-edge platforms could intensify their pursuit of promising startups, potentially ushering in a wave of consolidation. This year's deals include GSK, who acquired Elsie Biotechnologies in June 2024.
Restructuring
The adoption of AI in drug discovery is prompting a wave of restructuring and process reengineering across the pharmaceutical sector. Big pharma companies are reshaping their operations, integrating AI into every aspect of the drug development pipeline.
GSK exemplifies this shift. Since 2019, the company has deployed AI across its value chain, positioning itself as a leader in integrating machine learning into drug discovery. It was among the first to develop and train its own large language model, a foundational AI tool designed from scratch to handle the complexity of pharmaceutical data. The company's Onyx platform, a suite of next-generation AI systems, exemplifies this effort, enabling the use of proprietary genomic datasets to train machine learning models for discovering new medicines.
Beyond discovery, AI is embedded in GSK's operational processes. The company uses the technology to design clinical trials, ensuring greater efficiency and precision by stratifying patient populations based on advanced predictive analytics. Even the data generated from its genomic studies are managed by AI.
Real estate must adapt to support restructuring efforts, providing flexible and scalable solutions that align with evolving operational demands and strategic priorities.
Conclusion
While challenges persist, integrating AI into drug discovery is a seismic shift reshaping the pharmaceutical industry, particularly big pharma. From streamlining research pipelines to fostering unprecedented collaborations between biotech and tech giants, the impact of AI is reverberating across operations, talent strategies, and real estate decisions. With its combination of academic excellence and thriving AI and life sciences clusters, the UK is poised to lead this transformation.
[1] https://www.genengnews.com/gen-edge/the-unbearable-cost-of-drug-development-deloitte-report-shows-15-jump-in-rd-to-2-3-billion/
[2] https://www.asbmb.org/asbmb-today/opinions/031222/90-of-drugs-fail-clinical-trials
[3] https://www.morganstanley.com/ideas/ai-drug-discovery
[4] https://www.pfizer.com/science/focus-areas/rare-disease#:~:text=There%20are%20over%207%2C000%20known,one%20or%20more%20approved%20treatments.
[5] GlobalData
[6] PitchBook and Knight Frank Research
[7] LinkedIn and Knight Frank Research